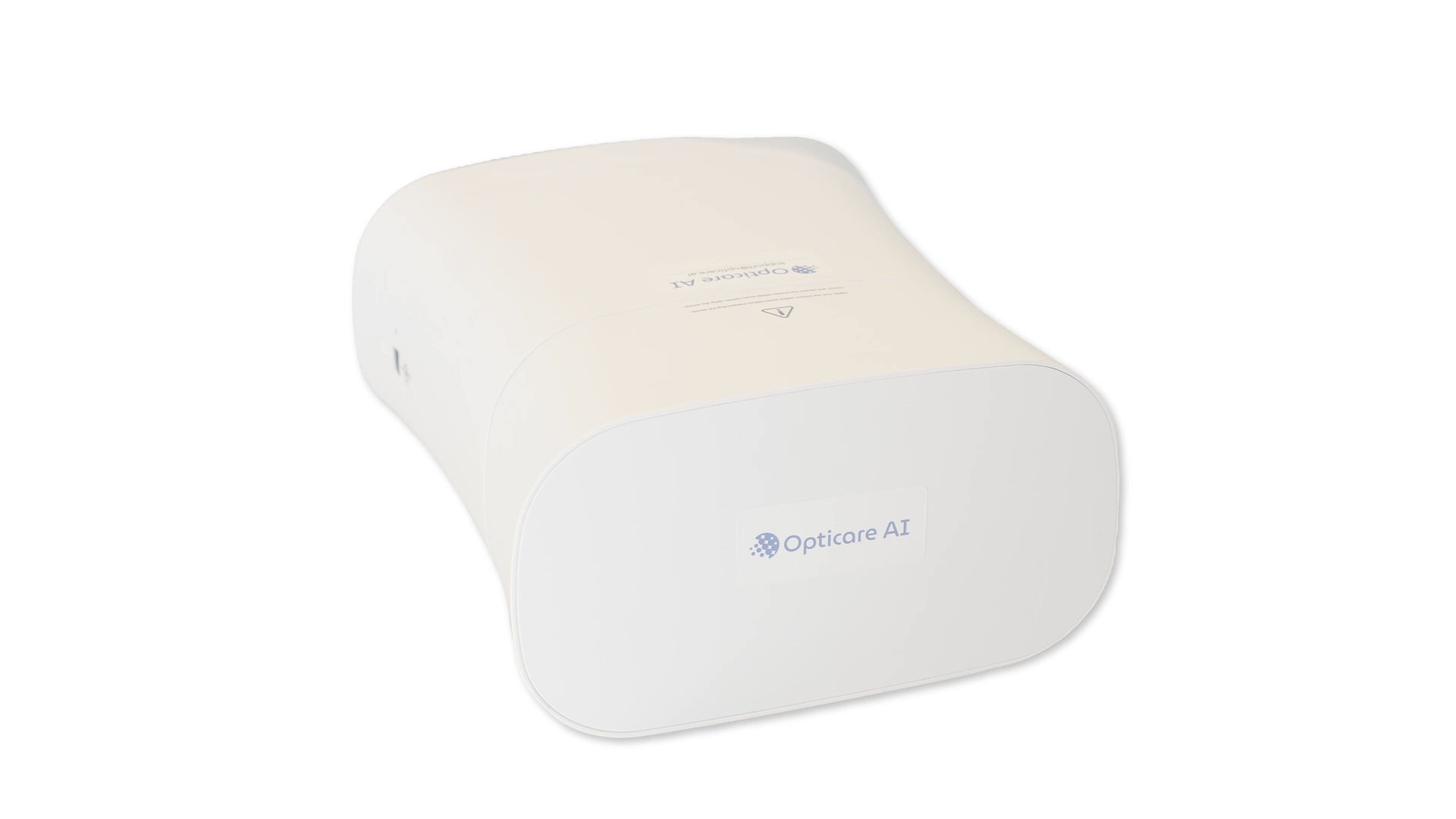
General Papers
Top studies that demonstrate the overall technology
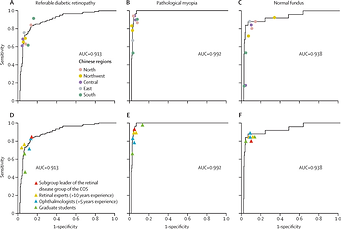
​
💡 This is the key paper: trained on 200K fundus photos and validated on externally-tested 18K photos from clinics across China. The study was sponsored by Sun Yat-sen University and pre-registered at Clinicaltrials.gov.
​
The dataset was further validated on another 11K public domain images (Kaggle) from EyePACS made up of Hispanic (50%) as well as White, Black, and Asians.
​
The mean AUC for identifying the 14 retinal abnormalities and normal fundus was 0·955 (SD 0·046) in the internal validation set and 0·968 (0·037) in the external test set.
​
Retinal Age

Retinal age gap as a predictive biomarker for mortality risk
​
Zhu Z, Shi D, Guankai P, et al Retinal age gap as a predictive biomarker for mortality risk British Journal of Ophthalmology 2023; 107: 547-554.
​
Based on a study of 80K fundus images from the UK Biobank, 2006-2010. Results suggest overall mortality risk goes up 2% for each year increase in your retinal age. Up 3% for non-cardiovascular and non-cancer death.
​
Simon Nusinovici, Tyler Hyungtaek Rim, Marco Yu, Geunyoung Lee, Yih-Chung Tham, Ning Cheung, Crystal Chun Yuen Chong, Zhi Da Soh, Sahil Thakur, Chan Joo Lee, Charumathi Sabanayagam, Byoung Kwon Lee, Sungha Park, Sung Soo Kim, Hyeon Chang Kim, Tien-Yin Wong, Ching-Yu Cheng, Retinal photograph-based deep learning predicts biological age, and stratifies morbidity and mortality risk, Age and Ageing, Volume 51, Issue 4, April 2022, afac065, https://doi.org/10.1093/ageing/afac065
A team of Singapore researchers trained on 120K fundus photos from 40K Koreans and evaluated against 56K UK Biobank participants.